Healthcare is undergoing a digital data explosion due to the utilization of electronic medical records, wearable devices, and the development of monitoring technology. This has sparked interest in using AI and ML to analyze these datasets for better healthcare outcomes.

A survey by AI in Healthcare discovered that over 40% of industry experts regularly use AI and ML.

The market is now valued in billions and set to grow significantly. AI and ML will help healthcare professionals handle large patient data volumes for accurate diagnoses and personalized treatment plans, leading to revolutionary changes in disease detection and therapy.
Take a deep dive into this blog to learn how AI and ML are transforming the healthcare industry.
AI/ML in Diagnosis
Diagnosing diseases accurately is important in healthcare, as errors can lead to serious complications and prolonged recovery. Statistically, misdiagnosis contributes to 10% of patient deaths and 17% of complications, highlighting the need for improvement in diagnostic precision.
In that case, healthcare providers can enhance diagnostic accuracy by leveraging artificial intelligence (AI) and machine learning (ML) technologies. These advanced tools offer promising solutions to mitigate misdiagnosis and improve patient outcomes.
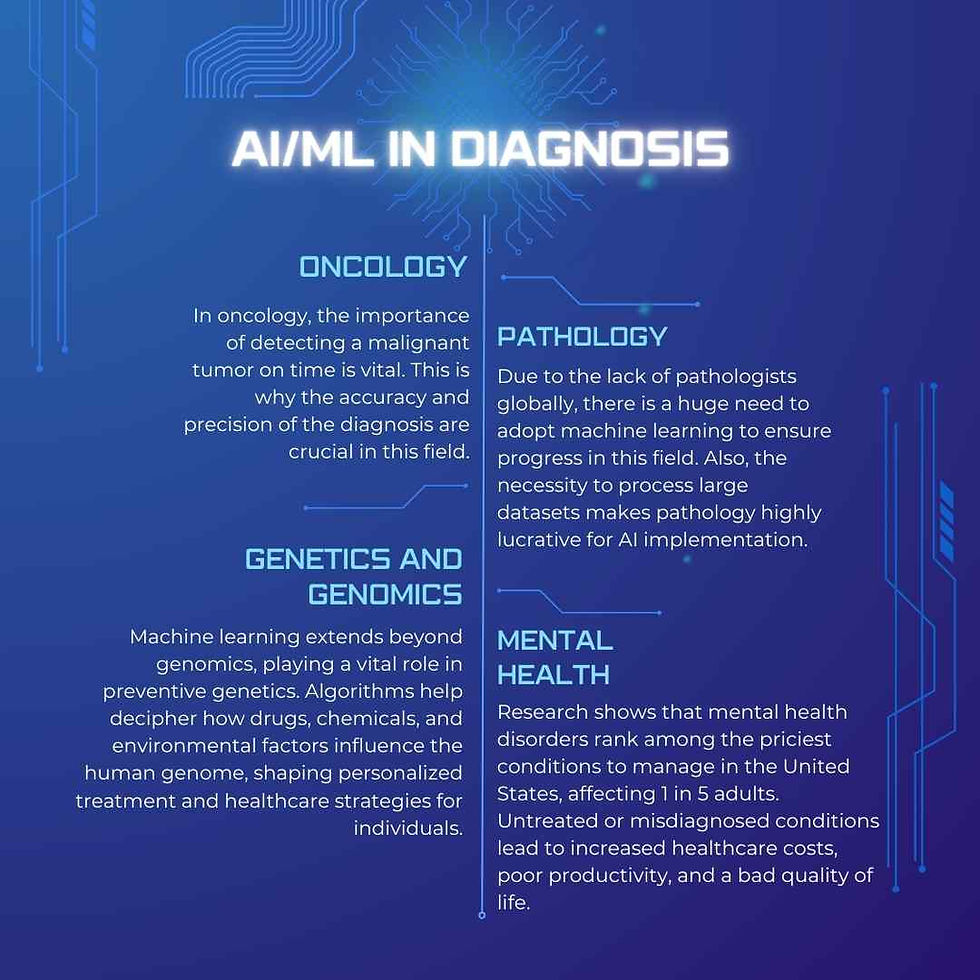
For better understanding, here are the top applications related to machine learning for medical diagnosis, demonstrating how these technologies can transform healthcare by improving diagnostic precision as well as decision-making processes.
Oncology
In oncology, the importance of detecting a malignant tumor on time is vital. This is why the accuracy and precision of the diagnosis are crucial in this field.
Detecting malignant tumors in their early stages is necessary in oncology, focusing on the importance of accurate diagnosis. Machine learning helps oncologists spot somatic mutations effortlessly.
Tools like DeepGene use machine learning algorithms to aid medical professionals in easily identifying somatic mutations, which are acquired changes in the genetic codes of cells. Artificial intelligence enables faster and more precise pinpointing of mutation markers compared to human capabilities.
When it comes to identifying the tumor, machine learning can precisely distinguish between malignant and benign ones within milliseconds.
Pathology
Due to the lack of pathologists globally, there is a huge need to adopt machine learning to ensure progress in this field. Also, the necessity to process large datasets makes pathology highly lucrative for AI implementation.
Here are some promising applications of machine learning in medical diagnosis:
Enhancing the accuracy of blood and culture analysis through automated quantification of tissues and cells.
Mapping disease cells and identifying critical areas on medical slides for further examination.
Developing tumor staging paradigms.
Boosting the productivity of healthcare professionals by accelerating profile scanning speed.
Genetics and genomics
Artificial intelligence is making gene research quicker and better, especially after the Human Genome Project.
Machine learning extends beyond genomics, playing a vital role in preventive genetics. Algorithms help decipher how drugs, chemicals, and environmental factors influence the human genome, shaping personalized treatment and healthcare strategies for individuals.
Mental health
Research shows that mental health disorders rank among the priciest conditions to manage in the United States, affecting 1 in 5 adults. Untreated or misdiagnosed conditions lead to increased healthcare costs, poor productivity, and a bad quality of life.
Artificial intelligence, specifically through machine learning, promises innovative advancements in mental health research and diagnosis efficiency.
Key applications include personalized cognitive behavior therapy (CBT) using chatbots and virtual therapists, preventive measures for high-risk groups against social isolation, and identifying and supporting individuals at risk of suicide.
Speed and Efficiency
AI and machine learning are driving advanced novel solutions and incredible progress to the healthcare industry – from early diagnosis to personalised treatments and disease development prediction.
They are helpful in analysing large datasets, uncover complex patterns and provide insights for informed decision-making, reducing medical errors and improving patient outcomes.
Provided below are the examples that demonstrate the speed and efficiency of AI/ML.
1. Computer Vision
Medical Imaging
AI technology can be used for medical imaging by analyzing images like skin lesions, helping classify them as cancerous or benign. Patients can easily upload images to a secure platform, where AI triages cases and helps with remote diagnosis, potentially reducing follow-up tests.
In another application, AI analyzes microscopic blood images, assisting in early disease diagnosis by counting blood cells precisely.
AI also analyzes X-rays and medical scans to detect conditions like cancer early, while also automating tasks like annotating images for treatment planning, thanks to tools like Facebook's Segment Anything Model (SAM), which speeds up medical experts' work.
Sports Analytics
AI can monitor athletes' fatigue levels by analyzing their performance and biometrics, helping identify potential injury risks. Trainers can then adjust training plans or take preventive measures to reduce injury risks based on these insights.
2. Text Analysis and Natural Language Processing
Information Extraction
AI can extract vital information, such as disease symptoms, from text or voice recordings. This benefits telemedicine by enabling doctors to evaluate patient data during calls. Also, it can automatically assign clinical codes to symptoms stated during online medical interviews, helping doctors save time on note-taking.
Recent advancements in large language models, like Google's Med-PaLM 2, show AI's ability to save medical knowledge and address accurate answers to medical questions. Such models perform at an expert level on medical exams, saving doctors' time for patient care rather than administrative tasks.
Text Classification
AI plays a crucial role in classifying medical data into categories such as treatment details, patient descriptions, and observed symptoms from medical histories. Also, it can be used in related areas such as medical invoice processing and classifying invoices for better management.
Sentiment Analysis
AI-powered sentiment analysis can be utilized in mental health apps, observing user sentiment during chats with doctors or bots. This analysis helps evaluate changes in mental health over time, enabling adjustments in therapy to better meet individual needs.
3. Machine Learning And Applied Data Science
Predictive Maintenance
AI-driven predictive maintenance in healthcare helps prevent equipment failures, decrease downtime, and ensure medical devices function well. This leads to improved patient outcomes and huge cost savings for healthcare facilities.
Sales Forecasting
AI facilitates sales forecasting in industries such as pharmaceuticals, helping in informed decision-making regarding product strategy. By analyzing huge amounts of data sets faster and more accurately, AI generates forecasts on the basis of historical data and market trends.
Simulation
AI can simulate various scenarios. It permits the generation and comparison of differet solutions.
For instance, AI can simulate infectious disease spread scenarios by utilizing mobility data along with disease models. This helps predict and mitigate disease outbreaks.
4. Recommendation Engines
Personalised medical therapy
A doctor could provide personalized treatment to a specific patient case if they had a complete health history of the patient. Another beneficial use of AI in personalised medical therapy is to customize medicine doses to specific patients on the basis of certain factors such as age, weight, and other characteristics.
AI has the potential to analyse data gathered by a wearable device, for example, a smartwatch, to find trends or patterns as well as alert doctors or patients about possible health risks.
Drug discovery and development
Recommendation engines can analyze a patient database to find suitable candidates for new drug testing based on clinical studies, genetics, and other factors.
AI can speed up scientific breakthroughs, as recently proved in the AlphaFold instance. The project's purpose was to build up a solution, the AlphaFold system, that could predict the 3D structure of a protein based purely on its genetic sequence.
It employs big genomic databases to predict protein structure and generates very accurate 3D representations of such proteins. It's a groundbreaking achievement because it allows us to better understand the protein's form and role in the cell, allowing us to build medications that work better with the protein's specific shape.
5. Augmented Reality
Medical Training
The AI-powered Augmented reality can be used to imitate medical procedures, allowing medical trainees to practise surgical methods in a realistic but low-risk setting.
The simulation can offer insight into technique, accuracy and timing. AR is expected to become a popular and important tool in medical education sooner.
Challenges of Adopting Machine Learning in the Healthcare Industry
The extensive use of innovative technologies such as AI/ML brings various challenges that can not be avoided.
They are:
Biases in Dataset
In any industry, machine learning algorithms are trained on vast data. Without appropriate supervision as well as training, these algorithms can hold and propagate the biases contained within the dataset. This could result in various consequences specifically in the healthcare sector.
Safety of Patient
The decisions made by machine learning algorithms totally depend on the data it has been trained on.
Unfortunately, medical data isn't always as precise and consistent as it should be. The records have gaps, profiles have inconsistencies, and other issues. So, if the input data is unreliable or wrong, the outcome is likely to be incorrect, which can cause improper patient care, deteriorating their health or even causing death.
Privacy Concerns
Another significant problem of adopting ML in healthcare is the volume of data produced that comprises sensitive or private information. This needs the implementation of more security measures. As a result, it is crucial to work with a renowned ML and AI development company that can offer top-tier security choices and make sure that your customer data is properly managed.
Generative AI in Healthcare
The Global Generative AI in Healthcare Market was valued at USD 0.8 billion in 2022, and it is predicted to reach USD 17.2 billion by 2032. Between 2023 and 2032, this market is expected to have the highest CAGR of 37%.
Generative AI is a novel type of machine learning that generates data by learning the features of real data. Artificially created patient data has the potential to transform clinical research and boost patient privacy.
Generative has the ability to decrease the clinicians' administrative load by auto-masking tasks including clinical coding, referral letter preparation, and clinical consultation. The growing number of hospitals and the change in the healthcare sector are increasing the demand for generative AI.
Significant Applications of Generative AI
Generative AI techniques are widely used in disease diagnosis, drug discovery, and drug development. It is important to transcribe medical photos and documents, including prescriptions, for remote patient treatment.
Another important role in virtual nursing assistants by connecting with patients with the highly effective care and treatment. The virtual nursing assistant method could secure the healthcare sector USD 20 billion annually.
Image analysis is a prolonged process in the healthcare industry, the generative AI decreases the time needed for image analysis. It helps healthcare providers to do effective diagnosis of diseases. The machine learning algorithms analyse 3D scans in real-time, offering up to 1,000 times faster results.
The healthcare industry is investing more on Electronic Health Records (HER) as it digitizes. It improves access to healthcare providers during treatment and increases the effectiveness of telemedicine. Several government programs are making huge investment in the healthcare business, which has boosted R&D efforts and driven market growth.
The Future of AI/ML for Healthcare
The future of machine learning looks optimistic in healthcare. Regardless of some limitations, machine learning aids help in the resolution of existing healthcare issues and the prediction of future suffering, thereby averting outbreaks across the world. Furthermore, it helps to boost the patient experience, clinicians' medical practices, and pharmaceutical operations.
The technology is responsible for assessing hundreds of different data points, tailoring treatment regimens, forecasting risks and outcomes, and performing other functions. AI and machine learning in healthcare also provide early warning signs for illnesses such as seizures or sepsis, which require extensive analysis of extremely complex datasets.
In the next few years, experts will be able to improve pharmaceutical delivery by blending machine learning and nanotechnology. Machine learning in healthcare can also allow "virtual biopsies" and advance the emerging science of radiomics.
ML-powered solutions can help clinicians reduce risk during procedures by focusing on even the smallest treatment elements. Furthermore, programmed robots may assist doctors in the operating room in the future.
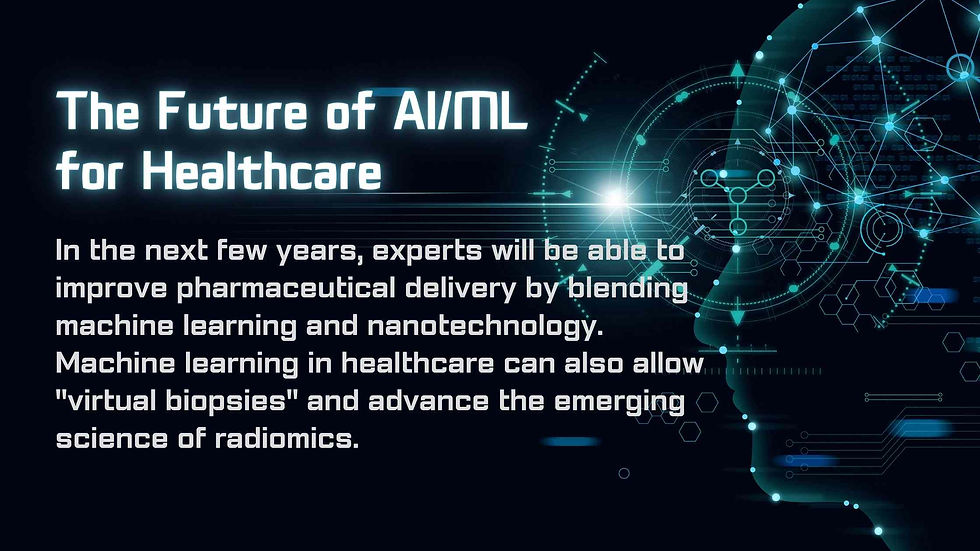
Machine learning solutions in healthcare can greatly benefit any doctor, researcher, clinician, or patient. Every day, technology makes a breakthrough, due to which a new application emerges that solves a real problem or establishes a milestone. The growth of ML in healthcare is steadily increasing, and it seems to achieve greater heights.
In Conclusion
AI and ML bring transformative changes in the healthcare industry, which include precise diagnosis and bespoke treatment as well as data-driven decision-making. As we navigate the intricacies and challenges, collaborations between healthcare providers, AI professionals, and regulatory bodies will drive innovation and assure responsible AI deployment.
We can envision a future of effective healthcare that is more accessible and equitable to all.
Opmerkingen